Build Design Systems With Penpot Components
Penpot's new component system for building scalable design systems, emphasizing designer-developer collaboration.
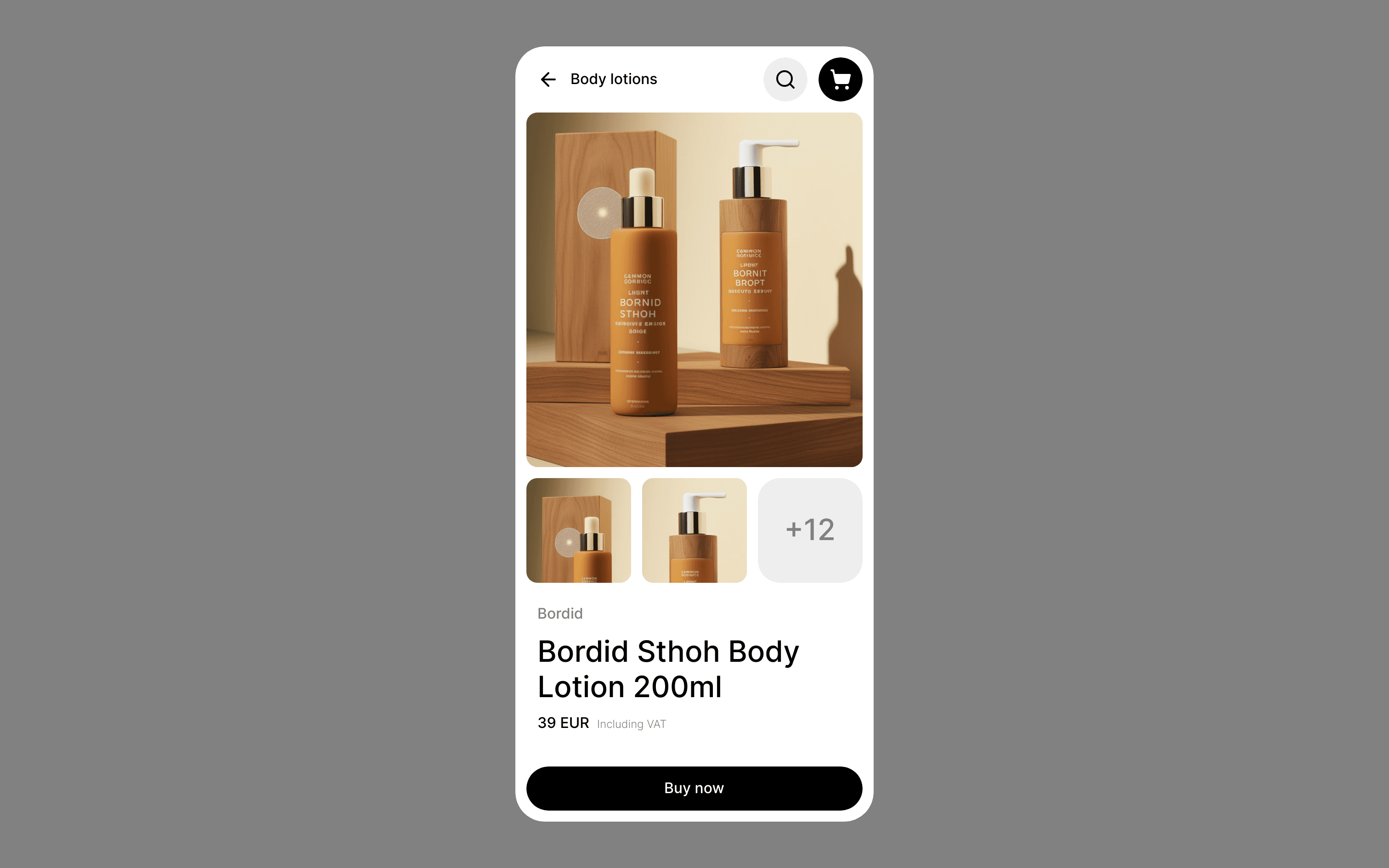
UX Planet — Medium | Anton Nikolov
I will share an interesting case that illustrates the selection bias very well. After that we can take a look at how to counter the selection bias when we make design decisions.
During World War II the British were loosing a lot of their bombers over Germany. They decided to add armor to them but it was expensive so they needed to choose carefully where to add it.
All bombers who had returned were documented well and all the areas of the damage they took were illustrated in diagrams. When the diagrams were overlaid the picture became clear. All the damage was focused around the wings and the body of the planes.
Diagram of the bombers which returned
The British first reaction was that they need to add armor to these areas.
However, they decided to ask for a second opinion. A Hungarian mathematician by the name of Abraham Wald was asked to take a look at the diagrams.
Wald’s data was based on the planes that had survived. The data did not include the bombers that were shot down.
His recommendation was the opposite to what the British thought was the solution. They needed to add the armor to the engine , the cockpit and the tail of the planes.
Hypotetical diagram of the bombers which did not return
Brilliant deduction from Abraham Wald! He resolved the problem with the bomber’s armor. He could have made a very nice designer. 🙂
It refers to the way data is collected and distorts our analyses and conclusions. This distortion can happen due to many reasons. Let’s take a look at 4 that we as Designers need to be aware of.
The most common reason is usually non-random sample. In the case with the British bombers that was the main cause for the selection bias(also in this case known as survivorship bias). They only had the data from the surviving planes and no data from the once that crashed.
The same is valid for digital design. Looking at data sample that is not based on random sample of your users will result in making a wrong decision.
Testing a product or a service in the wrong time interval or if the test is stopped prematurely can lead to selection bias.
Let’s say you’re running an A/B test. You need to run the test for minimum of 2 weeks if you want to see accurate results. Otherwise you are risking to distort the data, which will lead to selection bias.
Exposure of users to new variables during already running tests can also pollute the collected data. Influencing the users in this way can predispose them to act differently than they usually do. If the new variable is not included in the analysis of the data, your design decisions will be also influenced.
Cherry picking data that supports a specific position and excluding data that negate. It is a BIG no- no! Intentional or not, all of us should avoid creating evidence that is not representative of the truth.
Unfortunately, the web is a rich source of such information. It is easy to eliminate the issue of climate change when you cherry-pick only data that indicates flat or declining global temperature. Ignoring the holistic view/data will often come and stab us in the back.
When you’re collecting data you should collect it from all the samples.
For example, if you’re designing an app for the students in your university. You should collect data from all the students. All of them! If all students are represented in your analysis, there can be no selection bias.
Unfortunately, in most situations, it is not very practical to do this. Usually the target audience is too large or not available.
The key in these cases is random sampling. A random sample prevents selection bias. Make sure that you sample from the full set of things you have to generalize about.
For example, the bombers that returned were a subset of all the bombers. It seems, Abraham Wald knew that and made the right decision. The subset that was not available gave him the answer.
When you’re making the UI for your product /app make sure to collect the full data for your target audience. Avoid making generalizations based on subset of the data.
Be aware of the time intervals and exposure. Be critical, if these two causes for selection bias have influenced the collected data.
For instance, making A/B split testing around Christmas or other holidays could potentially distort the data and from there your decisions.
Cherry picking data that only supports your case is a big no-no!
Humans are pattern-discovering and pattern-making creatures. Our brains have evolved in a way that we automatically connect the dots.
In some situations this is super useful in others it can be misleading and lead to dire consequences. Only when we become aware of the biases, like the one discussed here, we can make better decisions.
Remember that good design decisions are based on well collected data.
If you found this article useful tap the ? and share so others can enjoy it, too.
Thanks a lot for your time!
Design principles: Selection bias was originally published in UX Planet on Medium, where people are continuing the conversation by highlighting and responding to this story.
AI-driven updates, curated by humans and hand-edited for the Prototypr community